

It combines the outputs of many weak learners to produce a powerful “committee” 6, 7. Boosting is one of the most powerful ML methods for selecting features and weight their predictive contribution to the classifier. NT1 vs NT2) when extracting hidden information from big and disparate data sources like the EU-NN database at machine scale, i.e., it frees from the limitations of human scale thinking. ML is specifically powerful in achieving classifiers (i.e. Unlike human knowledge machines will extract their own information based on an iterative information processing. Machine learning (ML) tools are becoming increasingly popular in medicine as these methods are able to detect patterns of symptoms and unveil information that is not visible for humans. One major goal of the EU-NN database is to identify distinctive phenotypes of central hypersomnias and to identify new biomarkers specific for these phenotypes. Currently this database includes 317 mixed types of variables per patient (e.g., categorical variables and discrete/continuous numeric variables from questionnaire data like features and history of cataplexy, laboratory data of multiple sleep latency test (MSLT) and polysomnography (PSG), biomarkers like CSF hypocretin levels and HLA DQB1*06:02) and 1380 patients from 26 centers with a number of missing data and multicollinearity, making it difficult to analyze with conventional analytics methods. Therefore, the European Narcolepsy Network (EU-NN), an association of leading European sleep centers, launched the first prospective European web-based database for narcolepsy and related disorders which allows collection, storage and dissemination of data on narcolepsy in a comprehensive and systematic way 1. For example, CSF-hypocretin measurement is not available in many centers. Even though international diagnostic criteria have been published, they may not be used in all countries. Third, the current international diagnostic criteria are based mainly on clinical experience but large multicenter international data are still missing. idiopathic hypersomnia or hypersomnias associated with psychiatric diseases), specific biomarkers are absent 5. In contrast, in narcolepsy type-2 (NT2) or other variants of central hypersomnias (i.e. Second, various forms of narcolepsy exist and only in narcolepsy type-1 (NT1, formerly referred as narcolepsy with cataplexy) low or absent cerebrospinal fluid (CSF) hypocretin levels serve as a specific biomarker 2, 3, 4. First, excessive daytime sleepiness (EDS), the key feature of narcolepsy, is shared by many other central hypersomnias and also a prevalent life-style consequence of insufficient sleep in our modern societies. The diagnosis of narcolepsy is challenging for several reasons. Narcolepsy is a rare central hypersomnia (CH) with an estimated prevalence of 0.02% in the European population 1. Our results suggest ML can identify features of CH on machine scale from complex databases, thus providing ‘ideas’ and promising candidates for future diagnostic classifications. mean rapid-eye-movement sleep latency of multiple sleep latency test contributes to classify NT1 and NT2 as confirmed by classical statistical analysis. While cataplexy features are recognized as the most influential predictors, machine find additional features, e.g. Stochastic gradient boosting, a supervised learning model with built-in feature selection, results in high performances in testing set. Here we apply ML to data from the huge European Narcolepsy Network (EU-NN) that contains hundreds of mixed features of narcolepsy making it difficult to analyze with classical statistics. Machine learning (ML) can help to identify phenotypes as it learns to recognize clinical features invisible for humans. Due to the considerable overlap of symptoms and the rarity of the diseases, it is difficult to identify distinct phenotypes of CH. Both types of narcolepsies belong to the group of central hypersomnias (CH), a spectrum of poorly defined diseases with excessive daytime sleepiness as a core feature.
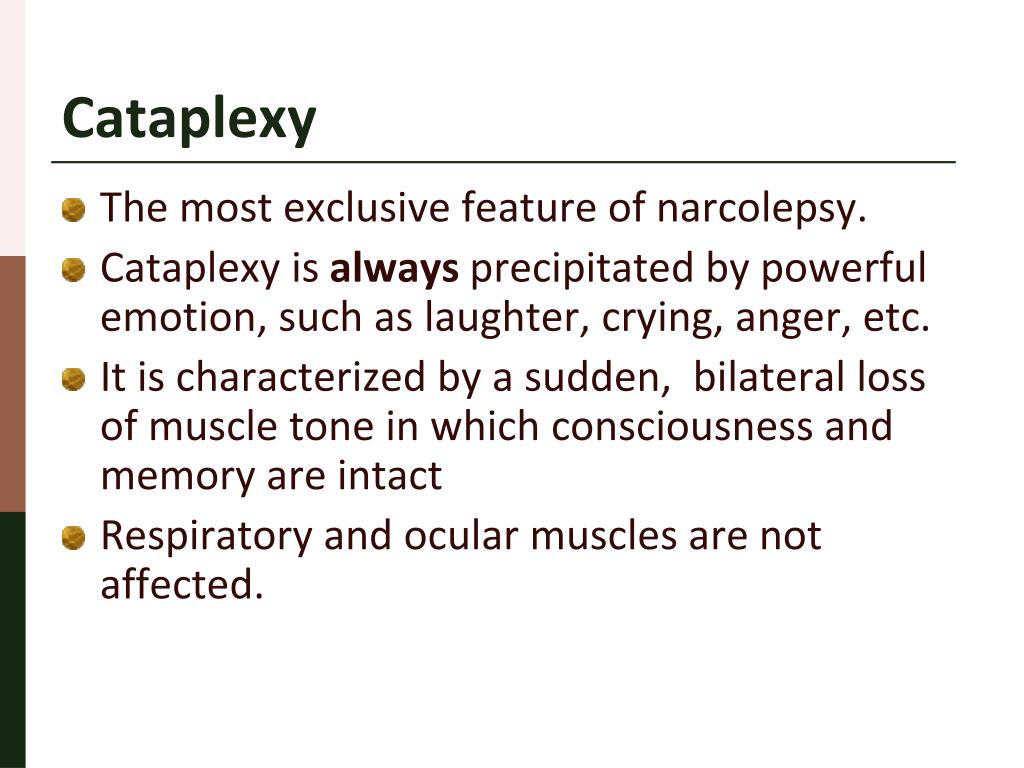
Narcolepsy is a rare life-long disease that exists in two forms, narcolepsy type-1 (NT1) or type-2 (NT2), but only NT1 is accepted as clearly defined entity.
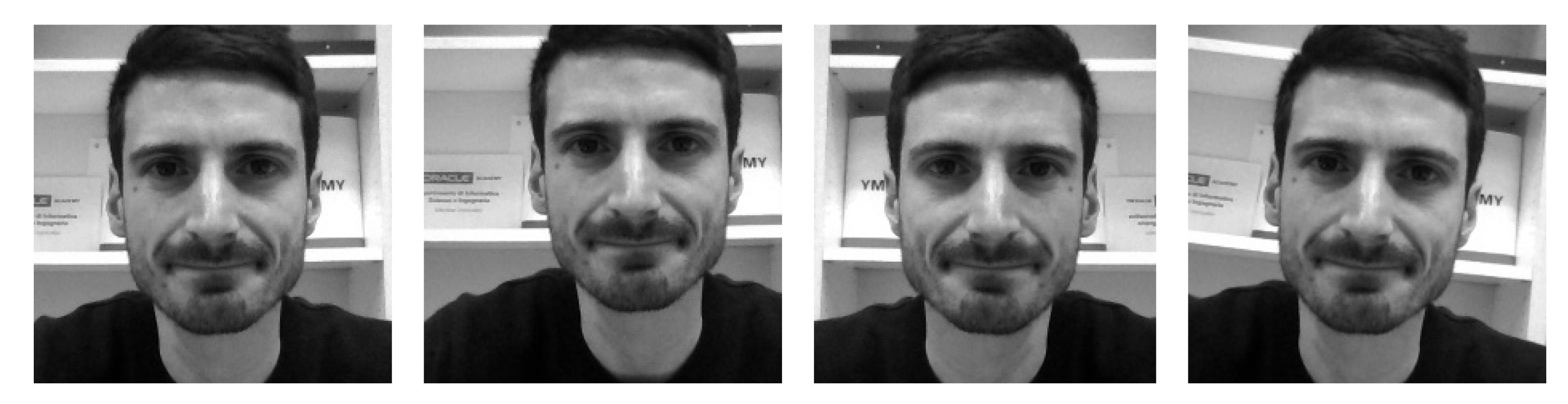
Scientific Reports volume 8, Article number: 10628 ( 2018) Exploring the clinical features of narcolepsy type 1 versus narcolepsy type 2 from European Narcolepsy Network database with machine learning
